This article will explain why machine-learning within AI needs more human input and how digital marketers can ensure algorithmic bias does not destroy marketing efforts.
We are living in an era where digital technologies and machine learning are evolving at a rapid rate. They are being used to make sense of an incomprehensible volume of data about people and the world we live in. Algorithms are making decisions that impact our lives and our future more than ever before. However, it is becoming more and more clear that this is not always being performed in a fair and transparent manner. In some instances, it is actually causing more harm than good.
What is AI?
IBM defines the term artificial intelligence (AI) as any human-like intelligence exhibited by a computer, robot, or other machine. Essentially, it refers to the ability of a machine to mimic the capabilities of the human mind – through experiential learning and examples, recognition, responding to language, making decisions and solving problems – and combining these with other capabilities to perform actions that a human might.
The AI ecosystem is complex. Put simply, think of AI as the universe of computing technology that features anything remotely resembling human intelligence. Within that universe there are machine learning and deep learning applications – models that involve algorithms which are built to make machines learn by themselves and make decisions just like we humans do, but at much greater speed and processing power.
AI is becoming part of our everyday lives and is transforming the digital marketing industry as we know it.
In 2020, Deloitte surveyed executives about their companies’ opinions and practices regarding AI technologies. They found that 67% of respondents are using machine learning today, and 97% were planning to use it within the next year.
Deloitte’s State of AI in the Enterprise, 3rd Edition
Machine-Learning Vs Human Intelligence
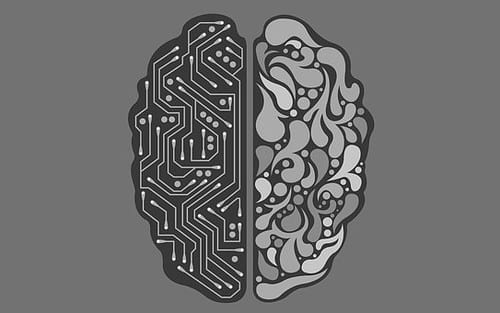
Machine and deep learning models rely on data inputs which they are then taught to analyse, identify hidden patterns, make classifications, and predict future outcomes.
IBM clarifies that while most machine-learning models are capable of supervised learning that requires human supervision – such as adjustment of the algorithms in the model, deep learning models are capable of unsupervised learning. This means that they can detect patterns in data with minimal human supervision.
This is where we must be cautious and not give AI too much responsibility just yet. Although machines may be able to imitate human behaviour to a certain extent, they lack ‘common sense’ and valuable human judgement. This can result in machine-learning algorithms within AI technologies to amplify and reinforce real-world bias.
How can AI Bias Affect a Marketing Strategy?
Let’s say a model is trained to predict future target markets. The data it is built with must be truly representative of those markets. Unfortunately, this is often not the case. Since the data sets used for algorithms are often extensive, the data used could consider factors that are not a true reflection of who the potential future customers are. For example, algorithmic bias in AI can be manifested as gender bias, racial prejudice and age discrimination. Such errors can cost you not only an entire marketing strategy but also reputational and regulatory damage.
When implementing AI and machine-learning tools, marketers must recognize and mitigate data biases early before they become detrimental to a strategy.
Data and AI Algorithm Bias in Growth Marketing, NoGood Growth Marketing Agency
How to Not Let AI Bias Destroy Marketing Efforts
The single most important thing digital marketers can do to mitigate the negative impact of AI bias is to constantly maintain the right level of diverse human involvement throughout the marketing chain. AI can harness remarkable automation and efficiency benefits in marketing efforts, but in its early stages of adoption human attention is crucial.
Marketers can reduce their vulnerability to AI bias in the following ways:
- Rely on as many different, complementary models as possible.
- Be mindful of where your data is coming from and check for misrepresentation in your datasets.
- Diversify your marketing staff and leadership so you can see where your own implicit biases come into play. Note that if the data we feed the machine reflects the history of our own unequal society, there is the risk of programming it to learn our own biases.
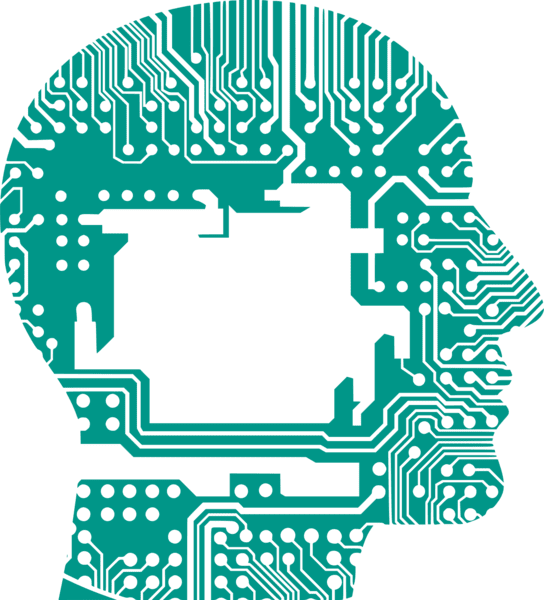
The AI Paradox
The paradox of today’s AI-driven marketing is that despite the promise of remarkable automation and other efficiencies from machine-learning, human intelligence remains paramount. Digital marketers must remind themselves that AI is first and foremost artificial. It’s value starts and ends with the input of both human observation and scrutiny.
References:
https://www.chiefmarketer.com/dont-let-ai-bias-derail-your-marketing-efforts/
https://www.ibm.com/cloud/learn/what-is-artificial-intelligence
https://www.marketingweek.com/ethics-of-algorithms/
https://nogood.io/2020/01/07/data-bias-ai-algorithm-growth-marketing/
Recent Comments